Decision making processes have come under severe scrutiny since the outbreak of COVID-19. Widely used forecasting methods did not take into account the possibility of a pandemic, meaning decision makers had not prepared to mitigate the resulting risks. These shortcomings highlight the need to adopt quickly Prescriptive Analytics. But to do so, what problems might you face, and how can you ensure it leads to more robust decisions?
What are Descriptive Analytics and Prescriptive Analytics?
Descriptive Analytics enables subject matter experts to generate insights by applying data exploration and visualization tools to historical data, using dashboards and business intelligence reports.
Predictive Analytics is a set of methodologies and tools that automatically identify patterns in historical data – whether internal to an organization or data acquired from an external source – and generate forecasts.
Prescriptive Analytics combines forecasts (predictive analytics) with mathematical optimization, operations research and decision sciences to identify the best course of action.
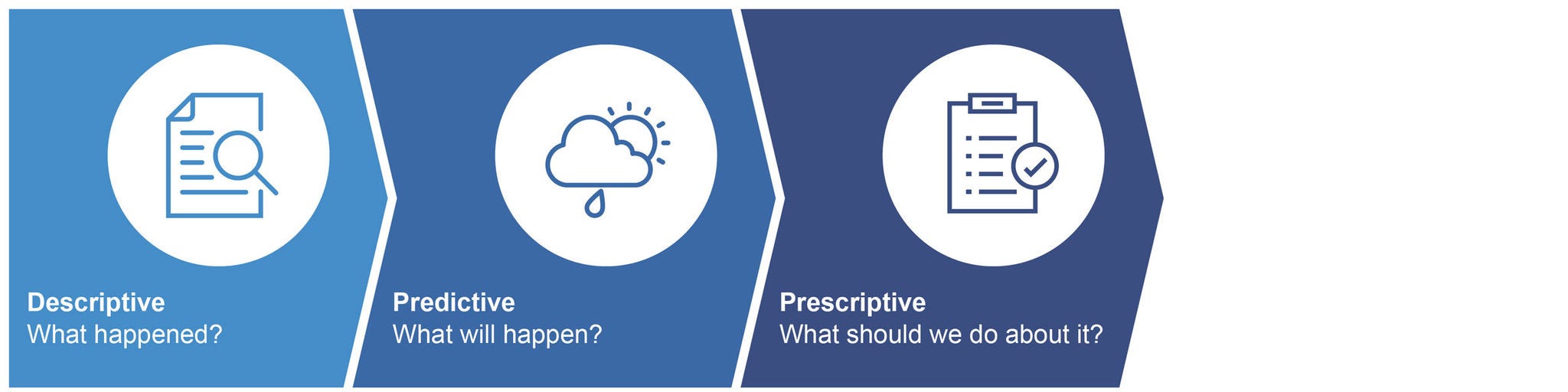
What are the main problems in adopting Prescriptive Analytics?
Improving decisions that are based on forecasts means addressing two problems: firstly, understanding the limitations of forecasting techniques; and secondly, getting better at using those forecasts to make the right decisions.
Problem 1: Forecasting models cannot predict the future
Forecasts generated on historical data by the most sophisticated Machine Learning models rely on strong assumptions and are not infallible. Let’s take a current example. Pandemics have occurred frequently throughout human history. They may be rare, but we know that they happen. Yet, it is fair to say that most companies did not anticipate the impacts of the COVID-19 outbreak.
This raises the question: could a mathematical model be expected to accurately predict the timing and extent of COVID-19? The answer is, probably not. But the truth is that forecasting systems employed across industries and functions, from supply chain management and distribution to finance, did not take at all into account the risk of such an event. This is largely because a pandemic on this scale has not taken place recently, i.e. in the timeframe which would be analyzed for the purpose of forecasting. Forecasting systems might likely not anticipate other major occurrences such as geopolitical risks or adverse weather events. Forecasts generated on historical data may be accurate only as long as the economic and competitive environment continues in line with how it is represented in past data. This is typically not the case.
Ultimately, predicting the future is impossible. Decision makers need to acknowledge this and deal with the uncertainty.
Problem 2: Reaching the right decision is hard even with the right forecasts
Even if you had infallible, 100 percent-accurate forecasts of the future, making the right decision would still be a challenge. In order to translate forecasts into decisions you need to formulate a decision process as a mathematical optimization problem. This is, in essence, Prescriptive Analytics.
In order to correctly specify a mathematical optimization problem, you need to set an objective function that expresses the goal that the company wants to achieve, and a set of constraints. A day-to-day example is route planning, where an algorithm defines the best path to travel from A to B. It can have multiple formulations with various sets of goals – such as wanting to minimize the distance travelled or minimize the travel time, and a set of constraints such as the road network, traffic density, speed limits, and construction sites.
It is hard to capture all the existing tradeoffs: While compiling a mathematical optimization problem might seem straightforward, defining an objective function and set of constraints that capture all possible trade-offs is extremely complex. Take production planning: as well as ensuring your resources are fully utilized, you must ensure there is enough time to carry out preventive maintenance – while guaranteeing fair employee schedules and taking individual’s vacation plans into account.
Lacking mathematical optimization skills: Successfully using Prescriptive Analytics also depends on having a broad set of methodological expertise available, including mathematical optimization techniques, operations research and decision science. In other words, people need to understand different kinds of math on top of machine learning and forecasting.
The required breadth and depth of knowledge is unlikely to be found in a typical business, though firms such as KPMG have built a range of such skills over many years, hiring professionals with vastly different backgrounds outside the standard data science curriculum.
How can you produce more reliable decisions?
The future cannot be predicted, so how can you cope with uncertainty in your forecasts? How can you manage risks that may not be reflected in your data and not recognized by your forecasting systems? The goal is to manage the risk of rare events to produce more reliable decisions as well as position your business to not only respond to disruption, but to take advantage of disruption to develop a competitive advantage. You can do this in three ways:
1. Applying probabilistic models and explicitly quantifying risks
Probabilistic models are an approach where uncertainty is explicitly quantified as a probability distribution: in probabilistic models, input variables are expressed as probability distributions; The output of a probabilistic model is also a probability distribution.
Probabilistic models provide a modelling framework that allows disruptive factors such as pandemics, adverse weather conditions and abrupt changes in the economic environment to be included. Rare events and catastrophe modelling become part of the day-to-day decision-making process, which in turn contribute to more resilient forecasts.
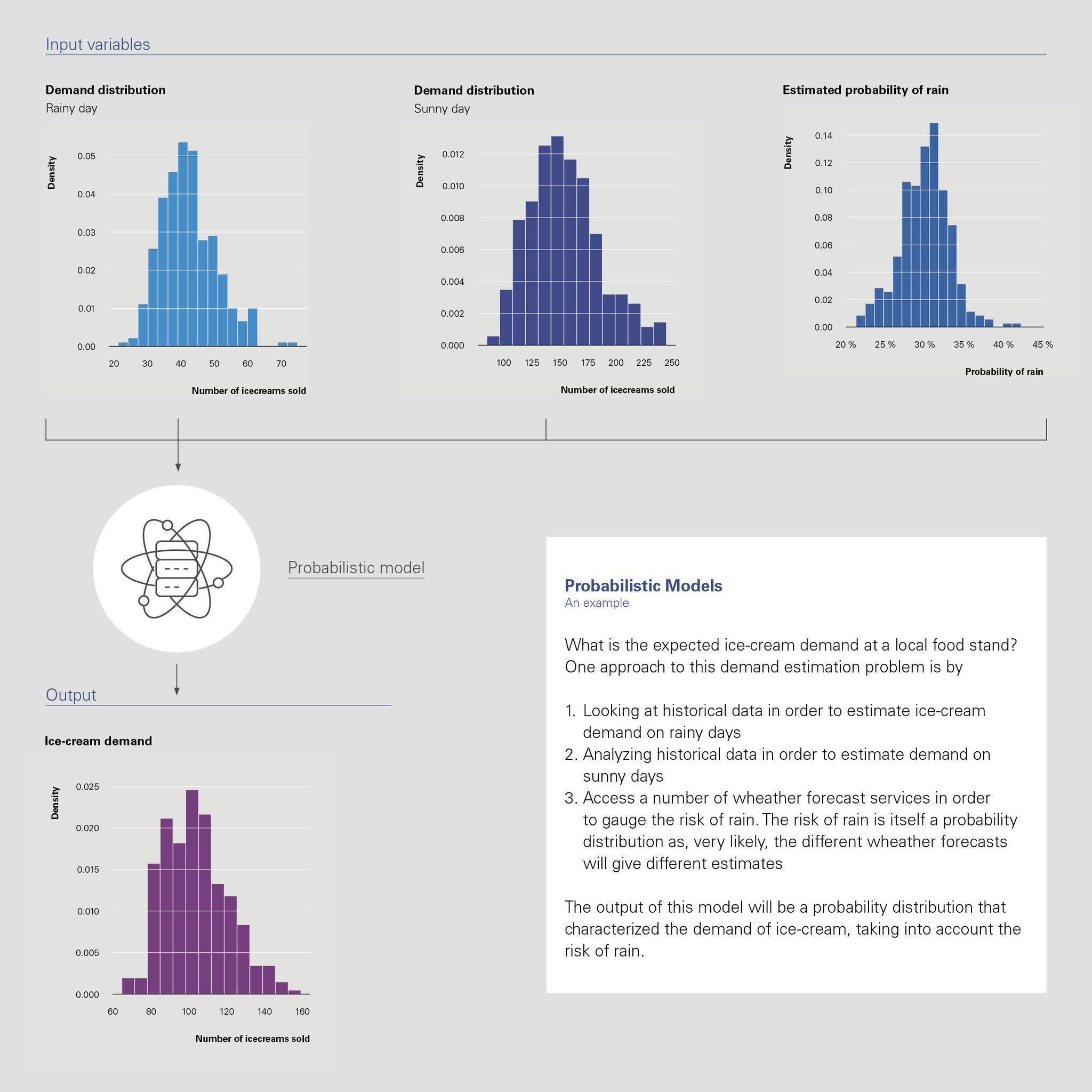
2. Enrich historical data with subject matter expertise
Key inputs can be estimated from historical data that you already hold or external data that you obtain from a third party. However, data might be biased or not paint the full picture. Create a systematic process to collect the knowledge that your subject matter experts (SMEs) have acquired internally and from conversations with clients or suppliers as well as from market surveys, competitive research or news articles. Such information can complement insights extracted from historical data.
When collecting the opinions of SMEs, it is critical to keep track of the sources and the degree of confidence SMEs have in their estimates. The greater the transparency in business assumptions, the greater the trust in, and adoption of, the Prescriptive Analytics solution.
3. Brainstorm about adverse scenarios and run stress-testing simulations
Probabilistic models allow rigorous simulations. By leveraging the knowledge of SMEs and brainstorming risks, you can develop scenario analyses that stress-test a business and go beyond the business and competitive environment reflected in the available historical data. Prescriptive Analytics models should generate optimal solutions that are robust to adverse scenarios. With scenario analysis, decision making evolves from simply extrapolating the past, to actively supporting continuous improvement and risk management.
Getting it right can deliver significant value
Prescriptive Analytics has the potential to boost profitability and long-term competitiveness by giving stakeholders the tools to make optimal decisions that are data-driven but also capture the knowledge of SMEs. Integrating scenarios analysis and catastrophe models leads to more robust and informed decisions, and therefore better quality outcomes.
As you consider how well your current models serve you, and how to strengthen your decision-making processes given the inherent uncertainties around us, consider the value of Prescriptive Analytics – and how you may use it to drive more value for your business.