Canadian businesses are starting to see the benefits of generative AI in higher levels of productivity and work quality, and many are looking at how to harness it to drive future innovation.
While generative AI can propel your business forward, it also leaves you open to risks that need to be addressed, such as the risk of leaking private data into the public realm. In a KPMG survey of generative AI users in Canada, 18 per cent of users revealed they had entered proprietary data about their company into a prompt.
Many other risks of AI aren’t obvious or fully understood. Yet, they could have major consequences for an organization, such as litigation, privacy violations, compliance violations, security threats, intellectual property theft, and even reputational damage.
For example, when content is produced by generative AI, there are risks around the ownership of intellectual property. The U.S. Copyright Office has determined that no one owns AI-generated content—neither the user who created the AI tool’s output by entering prompts, nor the creators of the tool itself.
These risks have led some organizations to ban access to publicly available generative AI platforms on company devices. But that won’t serve as a sustainable long-term risk management strategy.
We live in an era in which employees are encouraged to use their own tools and devices, whether in the office, on the road, or working from home. In a generative AI context, that means organizations will need to accommodate employee usage of generative AI in both a personal and professional capacity.
A KPMG survey found that generative AI adoption in the workplace is growing at an annual rate of 32 per cent. In practice, it’s difficult to clamp down on its use altogether—and punishing employees for using it can risk challenges around talent attraction and retention. It can also block productivity gains that your competitors may already be benefitting from.
Strategies for AI risk management
At the same time, our KPMG CEO Outlook survey found that Canadian CEOs are concerned about how AI will impact cybersecurity threats, privacy, misinformation, intellectual property, and bias in datasets.
Many find AI intimidating, and rightly so—it can be detrimental if misused or unchecked. Yet, avoiding or stalling its adoption could make your businesses obsolete in the near future.
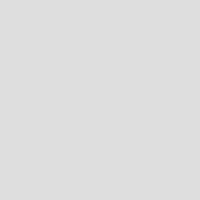
For risk leaders and the internal audit function, this calls for a careful risk assessment of generative AI impacts, so everyone thoroughly understands the risks of AI and the potential opportunities. Here are three critical AI risk areas for enterprises, and strategies that can help with AI risk management:
Risk area #1: Strategic alignment and oversight
Build a cross-functional governance committee:
In the context of generative AI, risk management isn’t just about technology. Risk touches on many aspects of an organization, which is why a wide range of stakeholders should be involved in the governance process.
Start by building a strong AI governance committee that is multi-faceted, with representation from across business functions, including legal and privacy, risk, technology, data, cyber and information security, and sales and marketing. This committee should seek external advice from AI experts, assist the C-Suite in making informed decisions about generative AI, and report to executive leadership to ensure trustworthy AI practices across the enterprise.
It’s also important to make sure everyone in the organization is educated about effective and responsible AI usage. The AI governance committee can help to build trust by mandating awareness and creating transparency around AI usage, including explanations of its workings, data usage, and decision-making processes.
Take an enterprise approach to AI use cases:
Various business functions are experimenting with generative AI and creating their own use cases. While experimentation is a good thing, developing siloed use cases can lead to long-term risk if they’re not part of a broader enterprise approach.
One of the greatest risks to an organization is lacking an enterprise view of AI risks and AI security concerns. If each department uses its own functional datasets, they won’t get a “single source of truth” across the enterprise. That could lead to a proliferation of inaccurate or inconsistent data. It’s also harder to understand the dangers of AI, monitor those risks, and build guardrails without a common frame of reference across business functions and units.
A common blind spot with generative AI is viewing its application through isolated silos instead of from a comprehensive enterprise perspective. Without this broader view, you risk diminishing the value you derive from generative AI.
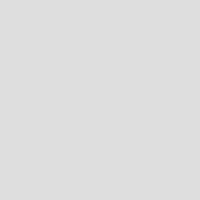
Risk area #2: Policies and procedures
Make sure company policies and processes keep up with generative AI:
Risk can be introduced through out-of-date polices and processes, such as those related to due diligence and vendor management.
For example, when engaging with a vendor, who’s looking at the T’s and C’s? With generative AI, you’re not dealing with traditional terms and conditions. By looking at the fine print, you might discover that a third-party vendor can use your proprietary data to train their models, which could be a security or privacy risk for your enterprise.
At the same time, many internal policies aren’t keeping up with the rapid technological advances of generative AI. For example, has your organization created an acceptable policy for internal and external generative AI tools used by employees? Risk leaders and the internal audit function will need to regularly revisit these policies and processes to keep pace.
Last, consider the minimum responsible AI standards you need to comply with, and what best practices you want to adopt. If your organization operates in North America and Europe, adopting a responsible AI framework and governance processes in compliance with the more stringent requirements of the EU AI Act may be in your best interest to protect your business and to prepare for upcoming Canadian regulations, such as the Artificial Intelligence and Data Act (AIDA).
Create risk categories for generative AI models that accelerate innovation:
While there should be a set of minimal controls in place as you’re building out models, not every model needs to be treated equally—doing so will quickly become a burden.
Risk categorization at the model level dictates the level of rigour and controls that should be in place for each model. While that may sound like a lot of extra work, the reason for putting AI risk controls in place is to accelerate—not to stifle—innovation.
Risk area #3: Third-party oversight
Mitigate the ethical risks of open source platforms:
Many organizations are using open source generative AI platforms, which can make it easier, faster, and cheaper to bring products and services to market. There isn’t a single tool that will meet all of your needs—and the tools are rapidly evolving—so integrating these tools into your IT environment will present new risks.
When using an open source platform, you’re also subject to the values and ethical controls of that particular vendor or platform. Are those values and ethical controls aligned with your organizational perspective? AI risk management will involve establishing ethical guidelines around data privacy, fairness, and accountability.
It will also involve fine-tuning models with your own guardrails, especially if you’re building a solution on a pre-existing platform. Some businesses are using a private cloud to train internal data so that data won’t accidentally get passed onto external models.
Building a trusted AI framework for your business
It can be challenging to build generative AI capabilities when there isn’t yet clear regulatory guidance or standards, though some progress has been made:
- The National Institute of Standards and Technology (NIST) with the U.S. Department of Commerce released a draft publication on April 29, 2024, based on the AI Risk Management Framework (AI RMF) to help manage the risks of generative AI.
- The European Union (EU) has approved the Artificial Intelligence Act, which establishes obligations for AI based on risks and impacts, dictates where organizations need to be more transparent about AI use, and provides guidance on risk categorization for models.
- While Canada is still awaiting the finalization of AIDA, which was tabled as part of Bill C-27 in 2022, a voluntary code of conduct was introduced in 2023 that provides guidance on the responsible development of generative AI.
This is a good start, but there are still missing pieces—and when it comes to generative AI, the technology is moving much faster than regulatory guidance.
While Canada, the U.S., and other countries will be coming out with similar guidelines and regulations, a trusted AI framework can maximize the benefits and minimize the risks, while building trust with stakeholders by aligning governance, safety principles, and AI ethics.
The key is embedding trust within your platform from the very start, during the proof-of-concept stage—rather than adding in controls after the fact. In other words, trusted AI fundamentals should be ‘by design.’
At KPMG, we’ve mapped international regulations against our Trusted AI Framework to come up with a set of best practices and considerations for implementing AI in a responsible way. The Trusted AI Framework is based on 10 key pillars: reliability, fairness, explainability, accountability, transparency, security, privacy, safety, data integrity, and sustainability.
Adopting a responsible AI framework and trusted AI principles that keep pace with the latest regulatory compliance measures can help you design, build, and deploy generative AI—and accelerate value with confidence.
Learn more about how our Trusted AI services are helping businesses accelerate generative AI with confidence.
Start with an enterprise AI risk management process
- AI Risk Assessment: Assess your current state and create a strategic roadmap to safely maximize your organization’s AI potential in accordance with professional, legal, regulatory and ethical guidelines.
- AI Governance: Review, establish, and monitor your governance frameworks, operating models, policies, and practices to support Trusted AI.
- AI Risk Monitoring: Test, examine evidence, and report on risk management processes, controls, and claims regarding the responsible use of AI technologies.
- AI Security: Build AI risk management and security plans, processes and tools to detect, respond to, and recover from cyber intrusions, privacy risks, software risks, and adversarial attacks.
- AI Development and Deployment: Establish robust risk management processes, controls and technologies to integrate Trusted AI into your end-to-end AI model management.
Insights and resources
Connect with us
Stay up to date with what matters to you
Gain access to personalized content based on your interests by signing up today
Connect with us
- Find office locations kpmg.findOfficeLocations
- kpmg.emailUs
- Social media @ KPMG kpmg.socialMedia