At CoP 28, global leaders set ambitious targets to scale clean energy rapidly. The declaration called for tripling renewable energy capacity and doubling energy efficiency improvements by 2030. The UNFCCC highlighted this as the “beginning of the end” for fossil fuels. However, the transition is proving challenging. Despite significant growth in renewables over the past decade, fossil fuels still dominate, as shown by the EI Statistical Review of World Energy 2024.
The Transformational Role of Contemporary AI
The advancement of AI is crucial in this context. Modern AI influences various scientific activities, from materials and equipment design to production and supply chain management. In contrast Classical AI primarily relies on rule-based systems and symbolic reasoning. These systems used to have limited learning capabilities and could not improve or adapt based on new data unless explicitly programmed to do so. The applications were largely deterministic, meaning they produce the same output given the same input. Modern AI leverages machine learning (ML) and deep learning (DL) techniques, allowing systems to learn from data and improve over time without explicit programming. The new age systems often use probabilistic models, which can handle uncertainty and provide predictions with varying degrees of confidence. With the advent of Generative AI (Gen AI), modern AI can create new content, such as text, images, and even music, based on learned patterns from existing data. It can also help produce new drug molecules at a fraction of costs, improve weather forecasting capabilities radically, assist in resilient cropping, reduce water consumption and much more. The practical applications are many, as is now becoming apparent in the sustainability sphere.

AI in Renewable Energy
AI’s contribution to the energy transition hitherto has been limited to specific, niche use cases. However, with the advent of GenAI, new possibilities are emerging. The renewables sector is increasingly integrating AI/GenAI for enhanced efficiency, optimization, and sustainability. AI and GenAI tools provide significant advantages over traditional management methods, from predicting optimal energy production times to optimizing grid distribution and managing energy demand.
Stages of AI Expansion in Clean Energy
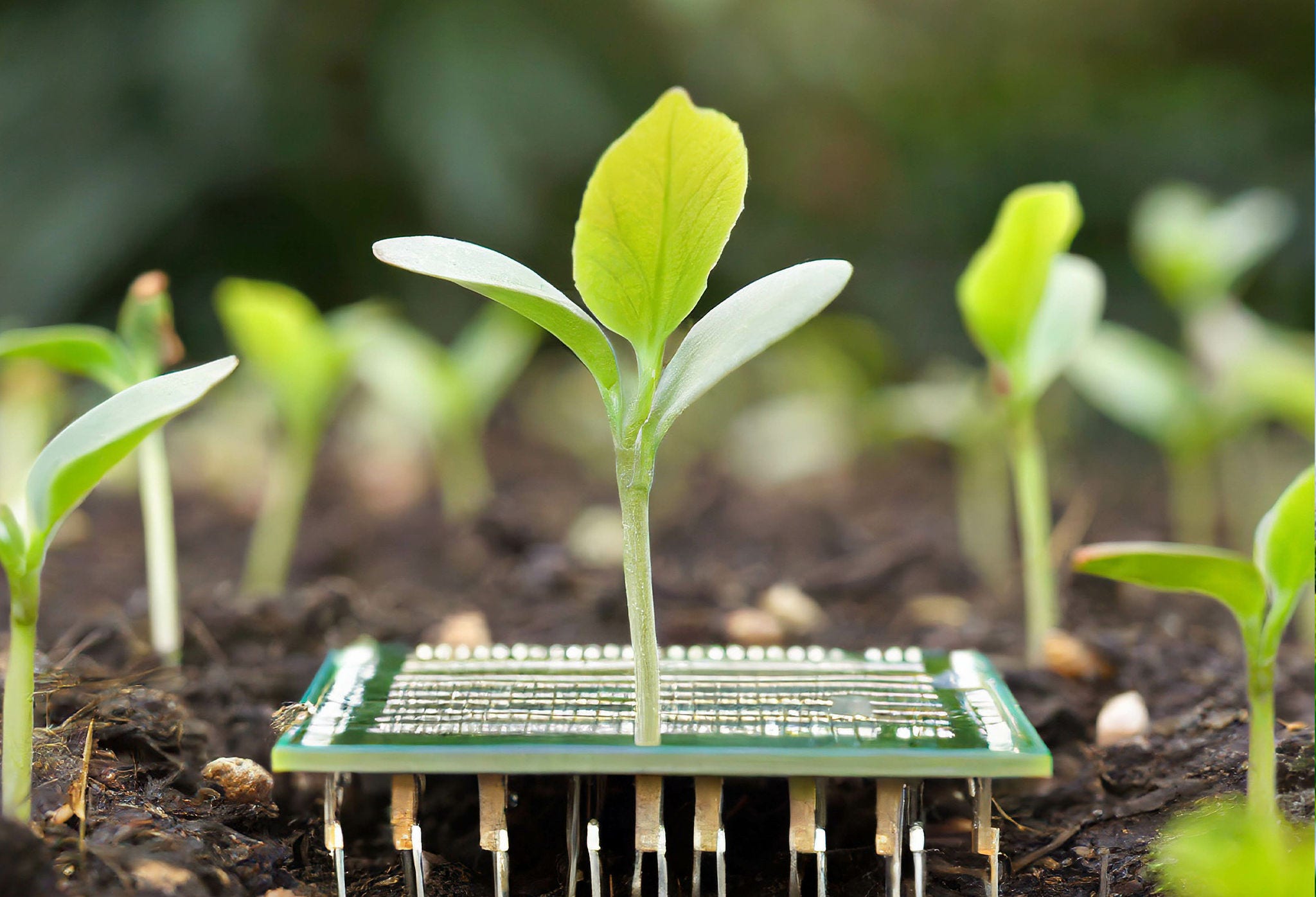
Initial applications included predictive maintenance for wind turbines and solar panels, enhancing efficiency and reducing downtime. AI algorithms dynamically adjusted turbine blade angles and solar panel orientations, highlighting AI’s capabilities for cost reduction and performance improvement.
As renewable adoption grew, AI’s role in optimizing energy production via predictive analytics for demand, supply, and pricing forecasts escalated. AI enhanced grid management, balancing supply with demand, leveraging decentralized grids, and optimizing energy storage, improving grid resilience and stability. AI also led to the discovery of efficient materials for solar panels, batteries, and wind turbine components.
GenAI marked a significant shift, generating novel solutions, designs, systems, and code. In renewable energy, GenAI enables advanced wind turbine design, supply chain management, optimal solar panel arrangement, and innovative energy storage solutions. GenAI accelerates innovation in renewable energy R&D by exploring vast design areas and options.
The combination of GenAI, QC, and HPC can solve complex energy sector problems. Modern AI enhances efficiency and sustainability by integrating renewable energy, optimizing energy grids, and improving reliability. QC offers unprecedented computational power for complex simulations, aiding in the development of efficient energy storage and conversion technologies. HPC provides the computational backbone for processing vast amounts of data and running complex simulations, including climate modeling. Together, these technologies offer powerful solutions for enhanced energy efficiency, advanced materials, and resilient energy systems.
Challenges and Regulatory Frameworks
Despite the potential, challenges remain. Data security, privacy, and reliability are immediate concerns. AI in supply chains exposes significant cybersecurity risks, including system breaches and attacks on broader energy infrastructure. The rapid growth in demand for AI specialists creates a recruitment bottleneck. AI’s higher energy consumption compared to traditional computing poses challenges for its integration into energy-efficient systems. Concerns about accountability emerge when decisions based on AI models lack full understanding or control.
Regulatory frameworks for responsible AI deployment are essential but lag behind technological progress. Governments and regulatory agencies must evolve and establish precise directives for deploying AI applications. Stronger guardrails will be needed as AI applications become pervasive.
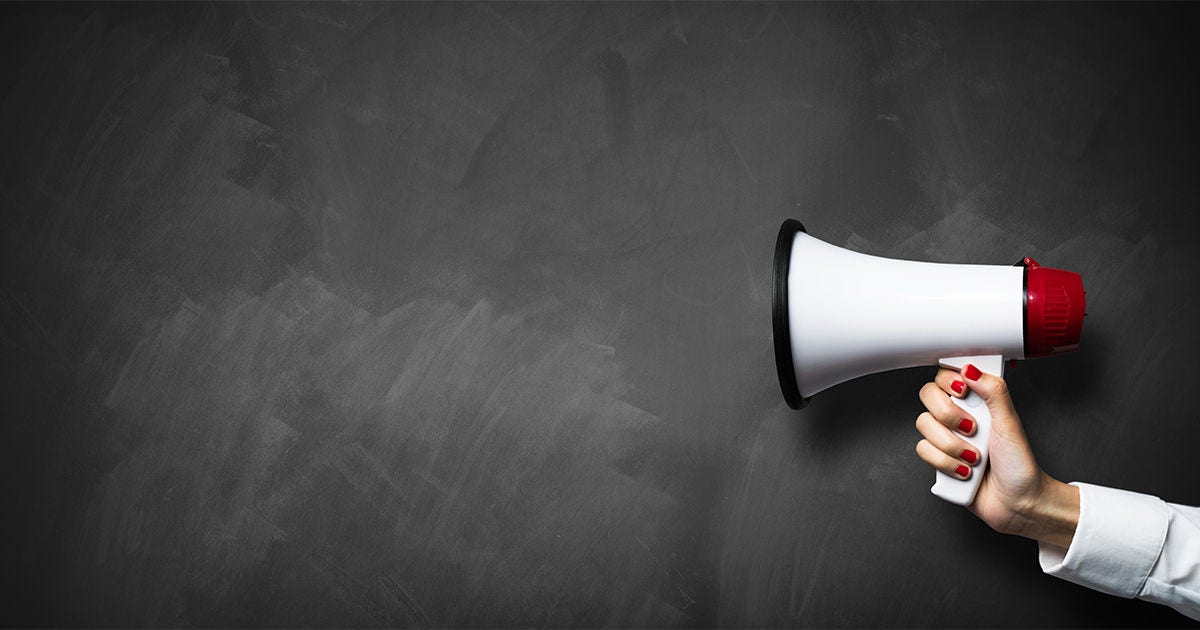
India’s Opportunity
India has a unique challenge in looking to grow its energy sector rapidly while decarbonizing simultaneously. This is a hard challenge since almost 90 percent of India’s energy needs are derived from fossil fuels1. As a major player in the global energy market, India must invest in trusted AI deployment for its energy sector. AI and Quantum will be demanding frontiers of technology. However, with its impressive track record in digital innovation and a vast talent pool, India can lead the way in these modern technologies. The country’s large market, significant carbon problem, and competent talent pool present a unique opportunity to pioneer and lead in AI and renewable energy.
In conclusion, AI, in conjunction with other technologies, opens up new possibilities for achieving the goals set out in CoP 28. While challenges remain, the potential for AI to enable the energy transition and create a sustainable future is immense. India is well poised for leveraging this new capability for advancing economic and climate goals in tandem.
A version of this article was published in The Economic Times - Energyworld.com. The same can be read here
[1] Statistical Review of World Energy, 2024
Author
Access our latest insights on Apple or Android devices
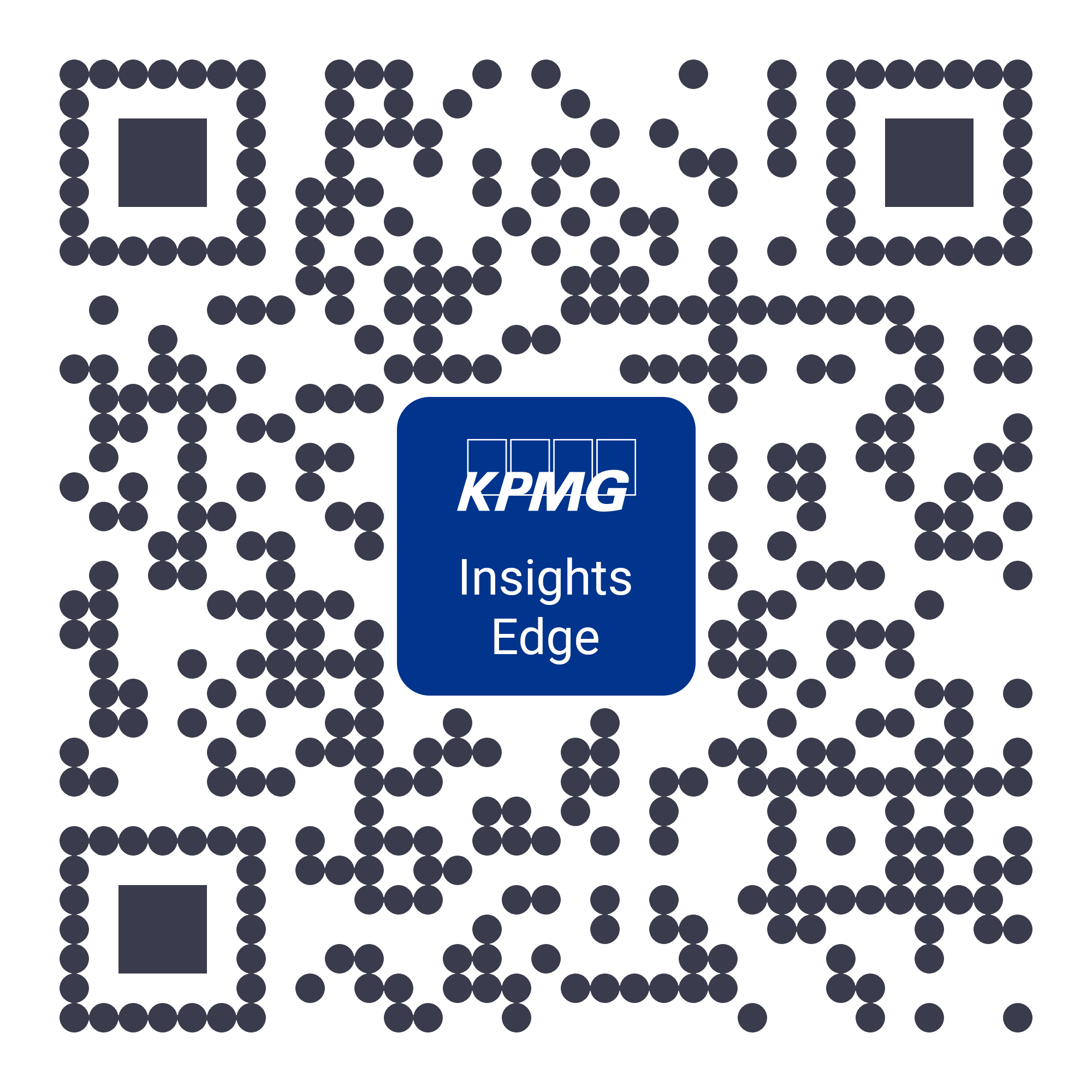