In the ever-evolving landscape of artificial intelligence (AI) (Figure 1) the phenomenon of hallucinating facts and figures has emerged as a perplexing challenge. Determination of the root causes behind this curious behaviour involves a deep understanding of the underlying technology.
Nigel Brennan, in our R&D Tax Incentives Practice delves into the intricacies of why large language models (LLMs) occasionally veer into the realm of the untrue, exploring the technical, ethical, and practical implications of this enigmatic occurrence.
What is a Large Language Model?
A LLM is a type of artificial intelligence system designed to understand and generate human-like language.
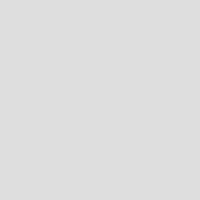
It is built on sophisticated neural network architectures, trained on vast datasets and can perform various natural language processing tasks, such as language translation, text completion, and answering questions. LLMs can comprehend context, infer meanings, and generate coherent and contextually relevant text based on the input it receives.
The Black Box of neural networks
LLMs are built on a type of neural network called a transformer model (Figure 2) and there is no way of working out exactly how these models arrive at specific conclusions. In other words, the output of an LLM is considered to be ‘Non-deterministic’.
This means that from the output, it is not possible to determine the input, meaning that detection of AI generated content can only be evaluated based on a margin of confidence, rather than a certain ‘true/false’ evaluation.
Figure 2: Transformer Architecture; the backbone of LLMs (https://medium.com/@ameemazainab/all-the-hype-about-finetuning-an-llm-llama-2-f204b78535d8)
In the absence of a concrete understanding of every learned parameter, the models may occasionally generate seemingly plausible information that is, in fact, a fabrication. This phenomenon, known as hallucination, occurs when the LLM extrapolates from its training data, creating information that appears accurate but lacks a factual basis.
Overfitting challenges
Hallucination in LLMs can be attributed, in part, to the challenges associated with overfitting. Overfitting occurs when a model becomes too closely tailored to its training data. As a result, the model may hallucinate information that aligns with the peculiarities of the training dataset.
For example, If the machine learning model was trained on a data set that contained mostly photos showing dogs outside in parks, it may learn to use grass as a feature for classification and may not recognise a dog inside a room. When faced with novel scenarios or inputs, LLMs may resort to generating responses based on superficial similarities to the learned data, leading to the production of inaccurate or hallucinated information.
Ethical considerations
The fine line between assistance and misinformation
The implications of LLMs hallucination extend beyond technical challenges, delving into ethical territory. As these systems become integral to decision-making processes in various fields, from healthcare to finance, the potential for disseminating misinformation raises concerns.
When an LLM hallucinates facts or figures, it may inadvertently contribute to the spread of false information (Figure 3), with consequences ranging from misinformation in news articles to inaccuracies in critical decision-making processes.
Figure 3: Oops. Source: https://www.sify.com/ai-analytics/the-hilarious-and-horrifying-hallucinations-of-ai/
Striking the delicate balance between providing assistance and avoiding the propagation of misinformation poses a significant ethical challenge for developers, researchers, and policymakers.
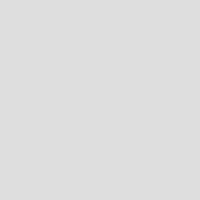
The quest for explainability and accountability
Addressing the issue of hallucination requires a concerted effort to enhance the reference-ability of LLMs. Researchers are exploring methods to make neural networks more interpretable, allowing stakeholders to trace the decision-making processes of these systems. Additionally, accountability measures must be implemented to ensure responsible development and deployment of LLMs.
The road ahead involves refining algorithms, establishing robust evaluation frameworks, and fostering interdisciplinary collaboration to create LLMs that not only perform functionally, but also uphold ethical standards. As we navigate the evolving landscape of LLMs, a deeper understanding of hallucination paves the way for more transparent, accountable, and reliable artificial intelligence systems.
This article originally appeared in Irish Tech News and has been reproduced with their kind permission.
Get in touch
If you have any questions on fine-tuning your AI systems, and avoiding hallucinations like those described above, contact Nigel Brennan of our Tax team. Our trusted AI and technology solutions deliver transformative results for your business.
We would be delighted to hear from you.
Discover more in Artificial Intelligence
4 Results
Nothing found